Experimental Imaging
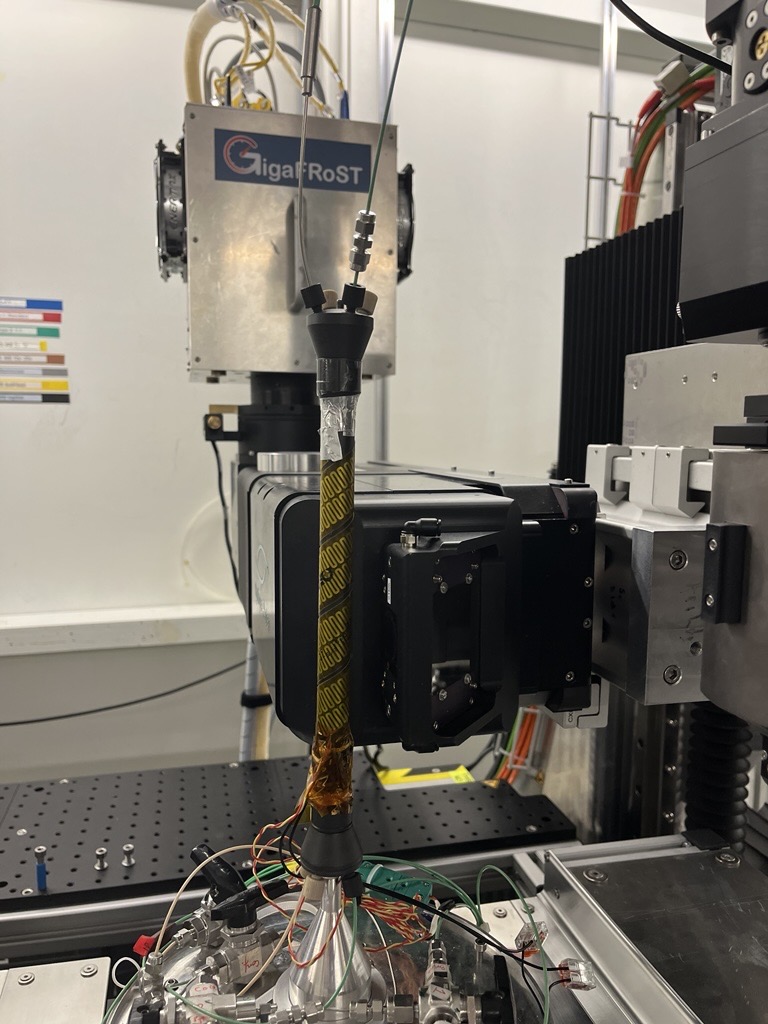
Multi-scale Imaging
At the DigiPorFlow Group, we capitalize on a range of imaging techniques to capture fluid flow processes across multiple length scales. Our in-house micro-CT setups allow us to non-destructively visualize pore structures in three dimensions, revealing fluid pathways and rock or material configurations in exquisite detail. To complement these capabilities, we also collaborate with specialized facilities for scanning electron microscopy (SEM) and energy dispersive X-ray spectroscopy (EDX). This partnership enables us to investigate the micro- and nano-scale features of porous materials—examining both their surface morphology and elemental composition. By integrating findings from micro-CT and SEM-EDX, we gain a comprehensive understanding of how fluids interact with the complex structures that govern flow and reactions in subsurface, natural, or engineered systems.
Reservoir Condition Experiments
At the DigiPorFlow Group, we operate a state-of-the-art facility designed to replicate the extreme conditions found deep underground. Our high-pressure, high-temperature (HPHT) experimental setups allow us to study fluid behavior in porous materials at the very conditions seen in geothermal reservoirs, hydrogen storage sites, and potential nuclear waste repositories. We also utilize custom reactors and effluent analysis capabilities to examine chemical reactions in real time, giving us a comprehensive view of how fluids flow, interact, and transform under subsurface conditions. By combining these advanced experimental tools with our broader research expertise, we bridge the gap between the lab and the field—driving impactful innovations in energy, environmental stewardship, and material science.
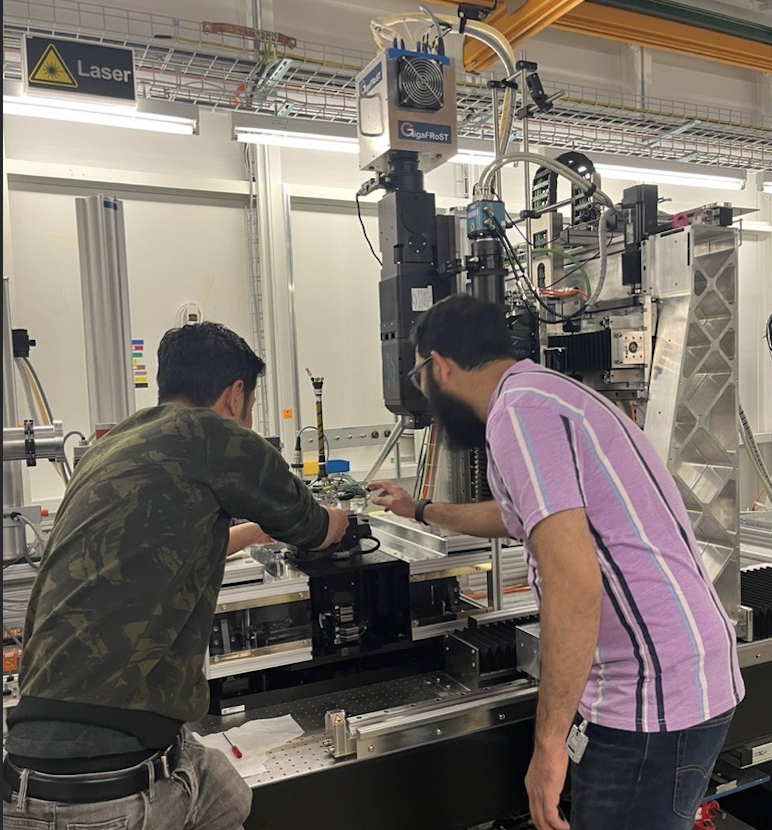
Synchrotron Imaging
At the DigiPorFlow Group, we harness the power of synchrotron imaging to capture high-resolution, real-time views of fluid flow within porous materials—from geological formations to bio-inspired structures. By using intense X-ray beams, we can visualize intricate pore networks and track fluid movement at unprecedented levels of detail. These insights are invaluable for advancing research in geothermal energy, hydrogen storage, carbon sequestration, and even nature-inspired designs, providing a clear window into the microscopic processes that shape our macroscopic world.
3D Printing and Microfluidics
Within the DigiPorFlow Group, we utilize advanced 3D printing technologies and specialized microfluidic platforms to replicate and manipulate fluid flow in custom-designed porous structures. By precisely fabricating materials with specific pore geometries, we can investigate fluid behavior under controlled laboratory conditions—mimicking both natural and engineered systems. Our microfluidics lab further refines these experiments, enabling us to visualize flow patterns and reactions at the pore scale. Through these complementary techniques, we gain critical insights into how fluids move, mix, and transform in complex networks, ultimately informing the design of more efficient, sustainable technologies.
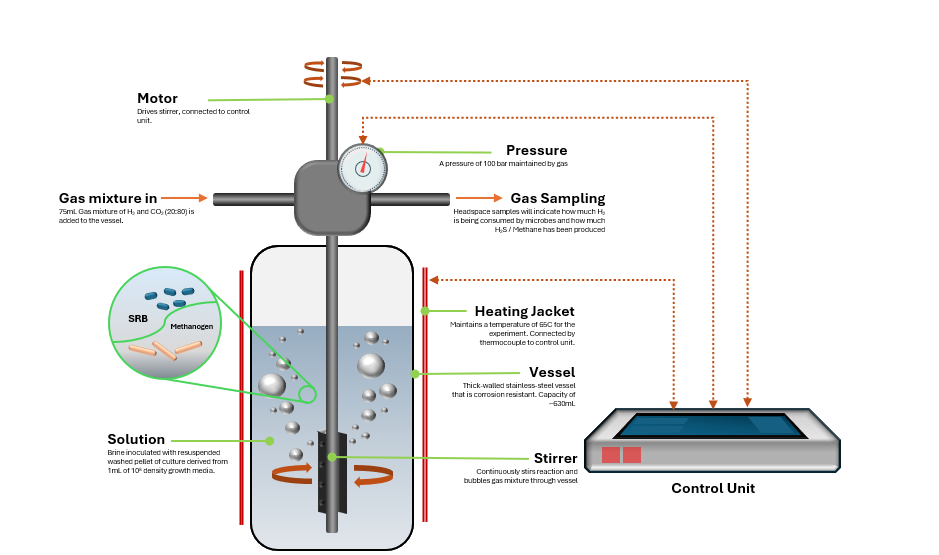
Subsurface Geomicrobiology
At DigiPorFlow we are pioneering new geomicrobiology capabilities to explore how microbes grow and interact in subsurface environments relevant to hydrogen storage. Our specialised biofilm reactors combined with our advanced imaging workflows enable the cultivation of diverse microbial communities in extreme environments, allowing us to study biofilm formation and microbial interactions within porous media. These innovative technologies facilitate detailed visualisation and analysis of microbial behaviour, enhancing our understanding of how microorganisms impact the stability and efficiency of hydrogen storage systems. Ultimately, our efforts provide critical insights for optimising hydrogen storage solutions and advancing sustainable energy technologies.
Open-source Modelling Software
The GeoChemFoam Project
The GeoChemFoam Project is the DigiPorFlow Group’s bespoke simulation platform, purpose-built to tackle complex geochemical reactions, heat, and fluid flow in porous media. Based on the open-source OpenFOAM framework, GeoChemFoam evolves through continuous development of new solvers and modules—expanding our ability to handle multi-phase flows, reactive transport, and heterogeneous geological structures. These specialised solvers capture processes like mineral dissolution, dispersivity, heat transfer, and fluid-fluid interactions, enabling precise modelling of real-world scenarios such as hydrogen storage, carbon capture, geothermal fluid cycling, and nuclear waste management. Our focus on solver innovation empowers researchers to dissect and optimise the intricate interplay of chemical and physical processes that shape subsurface systems.
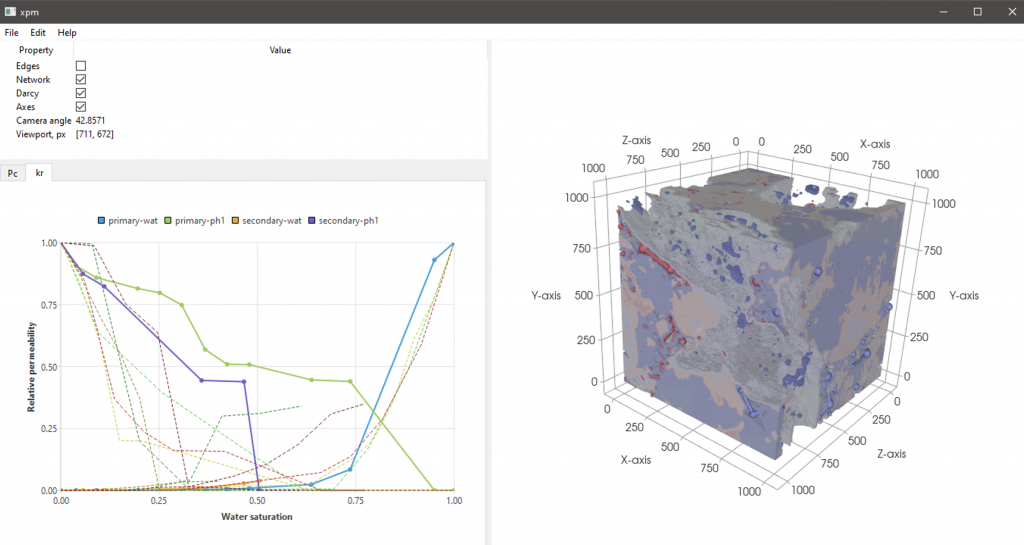
XPM: eXtensive Pore Modelling
XPM is a software for calculating absolute and relative permeability in multi-scale images. XPM combines traditional pore network modelling for resolved pores with Darcy-scale predictions in under resolved microporous regions. XPM is developed by Dr Dymtro Petrovskyy (Independent Consultant, Ukraine) in collaboration with the DigiPorFlow Group.
High Performance Computing with ARCHER2
Within the DigiPorFlow Group, we are pushing the boundaries of computational modeling by developing GeochemFoam on ARCHER2—one of the UK’s most powerful high-performance computing (HPC) systems. This enhanced platform enables us to run extremely large, detailed simulations that accurately capture the heterogeneity and complexity of real-world porous media. By leveraging massive parallelization, we can model coupled fluid flow and geochemical reactions at unprecedented spatial and temporal resolutions. Our work on ARCHER2, which is in collaboration with Dr Gavin Pringle at EPCC, helps us illuminate the intricate processes driving subsurface energy applications, carbon storage, and environmental management, setting the stage for truly transformative research and industrial innovation.
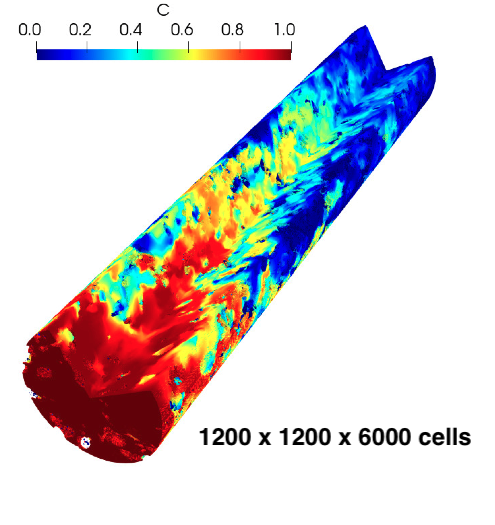
Machine Learning, AI, and Data Science Applications
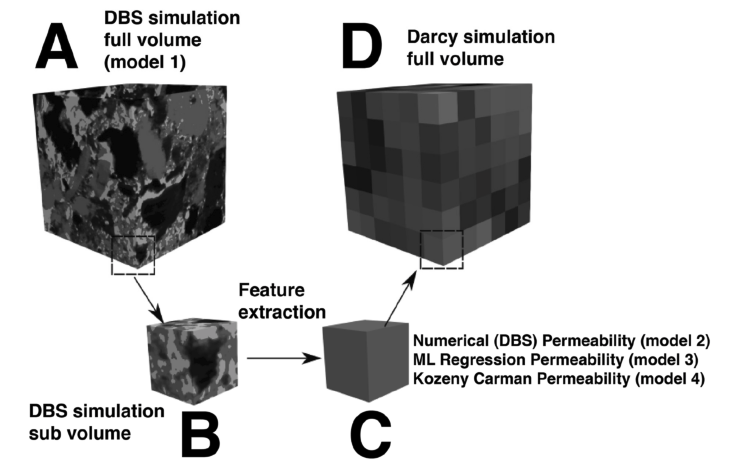
Data-Driven Prediction
Building on our extensive experimental and simulation datasets, we employ a suite of machine learning algorithms—ranging from deep neural networks to ensemble random forests—to capture nuanced relationships within porous media. For instance, micro-CT scans provide 3D pore-scale data that we pair with flow and reactive transport measurements. By feeding these large, high-dimensional datasets into our models, we can accurately predict flow behaviors under various pressures, temperatures, and chemical conditions. This data-driven approach empowers us to rapidly assess scenarios like carbon storage integrity or geothermal reservoir performance, reducing the need for time-consuming and expensive trial-and-error experiments.
Automated Image Analysis
A cornerstone of DigiPorFlow research is high-resolution imaging—micro-CT, synchrotron imaging, and collaborative SEM-EDX facilities. To process and interpret the vast array of images produced, we rely on computer vision and AI-based segmentation techniques. Our pipelines automatically identify pore spaces, fluid phases, and mineral grains, enabling us to track flow pathways and pinpoint reaction fronts with greater speed and consistency than manual methods. This capability is especially crucial when analyzing fast-evolving processes like geochemical reactions or multiphase flows under extreme pressures and temperatures. By automating image analysis, we can focus on interpreting results and designing innovative experiments that push the boundaries of porous media research.
Surrogate Modelling & HPC Integration
Running full-scale, high-fidelity simulations is computationally demanding, especially when modelling reactive flow through highly heterogeneous geological formations. To overcome these constraints, DigiPorFlow in collaboration with the AI4NetZero group develops surrogate models—lighter, data-trained approximations of our most complex numerical simulations. By integrating these AI-powered surrogates into our modelling workflows, we can quickly explore large parameter spaces, optimise processes, and home in on critical conditions without expending excessive computational resources. This hybrid approach of high-fidelity plus surrogate modelling offers a best-of-both-worlds solution, delivering both speed and detail in understanding how fluid flow evolves in challenging subsurface scenarios.
Advanced Data Science & Exploratory Insights
Beyond predictive modeling and image analysis, our group leverages a broad spectrum of data science tools—from dimensionality reduction methods (PCA, t-SNE) to advanced clustering algorithms. These techniques help us detect emergent patterns, anomalies, and correlations within multidimensional datasets that might be overlooked by traditional approaches. For instance, by combining data from microfluidic experiments, in-house HPHT reactor runs, and HPC simulations, we can reveal the interplay between structural heterogeneity and fluid flow at different scales. These exploratory insights guide the design of future experiments, inform more refined hypotheses, and ultimately catalyse breakthroughs in areas like geothermal energy optimisation, hydrogen storage safety, and sustainable building materials design.
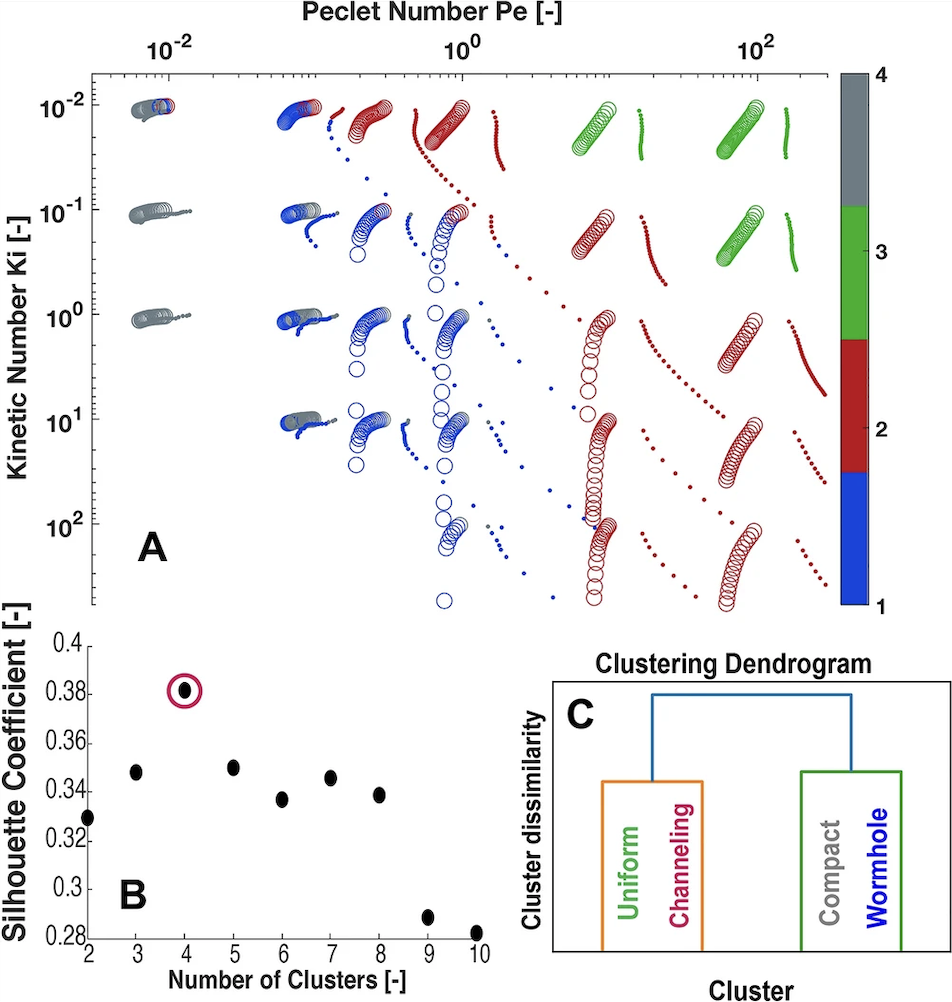
Sponsors
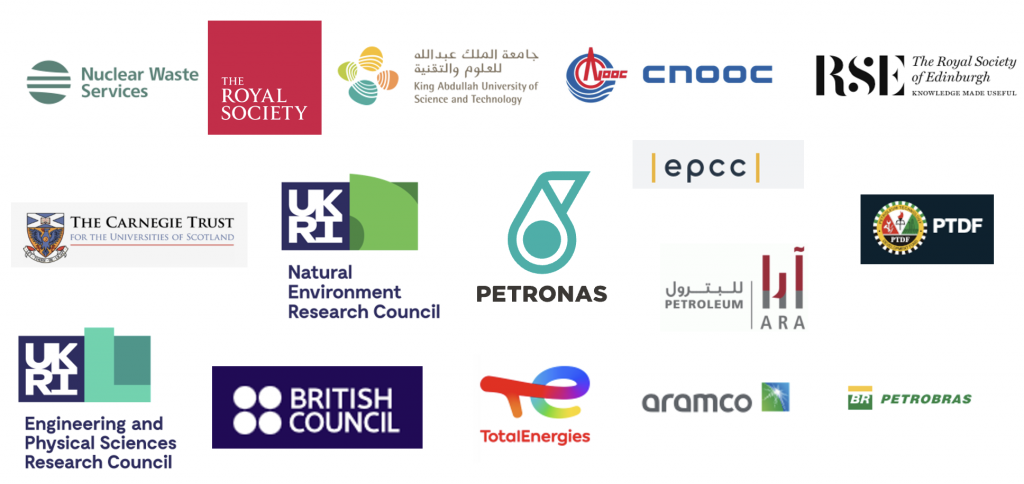